AI model helps identify medications to repurpose for rare diseases
Tool analyzes treatments for thousands of diseases
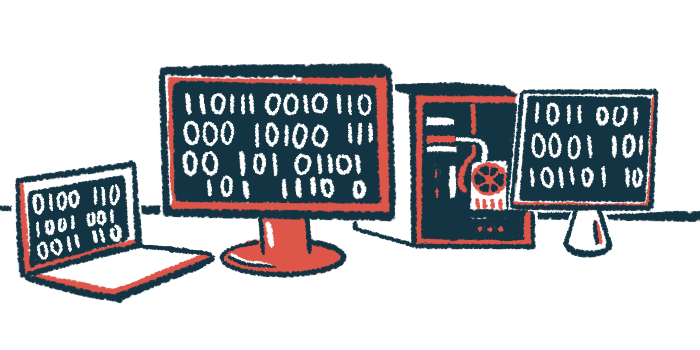
Researchers have developed an artificial intelligence (AI)-based model designed to identify existing medicines that could be repurposed to treat rare conditions such as cold agglutinin disease (CAD).
The model, called TxGNN, was able to identify possible therapeutic candidates for more than 17,000 conditions in a study, and researchers believe it has the capacity to be applied to additional diseases in the future.
Its developers are particularly hopeful that TxGNN will spur innovation in the rare disease space, as people living with many of those conditions don’t have any available treatments. To encourage clinicians and scientists to use the tool, they’ve made it available for free.
“When it comes to rare, ultrarare, and neglected conditions, we foresee this model could help close, or at least narrow, a gap that creates serious health disparities,” Marinka Zitnik, PhD, the study’s senior author and an assistant professor at Harvard Medical School, said in a university news story. Finding new uses for existing medications is “a faster and more cost-effective way to develop therapies than designing new drugs from scratch,” Zitnik said.
The findings were described in the study, “A foundation model for clinician-centered drug repurposing,” which was published in Nature Medicine.
Treatment development costly, slow
There are more than 7,000 rare diseases, among them CAD, an autoimmune condition in which self-reactive antibodies attack red blood cells at cold temperatures.
Despite the fact that rare diseases collectively affect more than 300 million people worldwide, treatment development remains lacking, with about 5%-7% of these conditions having a treatment that’s approved by the U.S. Food and Drug Administration (FDA).
Developing therapies and cures, and then bringing them to market, can take decades and cost billions of dollars. A promising approach for rare disease treatment development is to repurpose medications that are already used for other indications.
“The premise behind repurposing is that drugs can have [multiple] effects beyond the mechanism of action of their direct targets,” the researchers wrote.
Because these treatments are already used clinically and have established safety profiles, repurposing them can help expedite the development process for a new indication while reducing costs.
But there’s a need for more streamlined processes to do that. While nearly 30% of FDA-approved medications are later cleared for another indication, this usually happens by luck after years of patient observations or doctors prescribing such medicines off label.
“We’ve tended to rely on luck and serendipity rather than on strategy, which limits drug discovery to diseases for which drugs already exist,” Zitnik said.
Moreover, because there tends to be less knowledge about the biological mechanisms underlying rare diseases or conditions without approved treatments, it’s harder to match them with existing drug mechanisms.
The researchers’ goal was to develop a better tool to identify and rank promising therapies through AI and machine learning. Machine learning is a form of AI that uses algorithms to analyze data, learn from its analyses, and then make a prediction about something.
TxGNN contains a predictor component built using a large collection of data from more than 17,000 diseases, 92% of which don’t have any FDA-approved therapy. This includes information about cell signaling pathways, gene activity, and other disease characteristics and mechanisms.
The algorithm could extract that information, looking at shared features between diseases that are well understood and ones that aren’t, to predict which of nearly 8,000 different drug-repurposing candidates might be a therapeutic option for a given condition.
Drug-repurposing candidates included both FDA-approved medications and experimental therapies currently in clinical trials.
CAD was among the diseases included in the study. Some of the top therapeutic hits for the disease belonged to a class called corticosteroids, which have potent anti-inflammatory activity.
TxGNN also predicts if certain medications would be contraindicated, or not recommended due to the potential to cause harm, for specific diseases, or if they’d be expected to cause side effects in certain patient groups.
These are the types of insights usually learned by trial and error in early-stage trials. Predicting these factors ahead of time can help researchers hone in on treatments most likely to succeed once they reach clinical development.
TxGNN also has an explainer module that gives the medical rationale for why a treatment was selected as a candidate for a particular disease.
Compared with eight existing AI drug-discovery models, the tool was found to be, on average, nearly 50% better for identifying therapeutic candidates, and 35% more accurate for predicting contraindications.
Through a series of experiments, the team further validated their approach, showing that TxGNN’s predictions align with off-label prescriptions already used clinically as well as the medical opinions of human experts.
Still, “predicted drugs would need extensive screening to establish safety and efficacy and determine other drug parameters, such as drug dosage and the sequence and timing of treatments,” the researchers wrote.
They also noted that TxGNN may have broader applications beyond rare diseases.
“Even for more common diseases with approved treatments, new drugs could offer alternatives with fewer side effects or replace drugs that are ineffective for certain patients,” Zitnik said.